Suggested Future Trends in Computer-Aided Recognition Methods and Problems
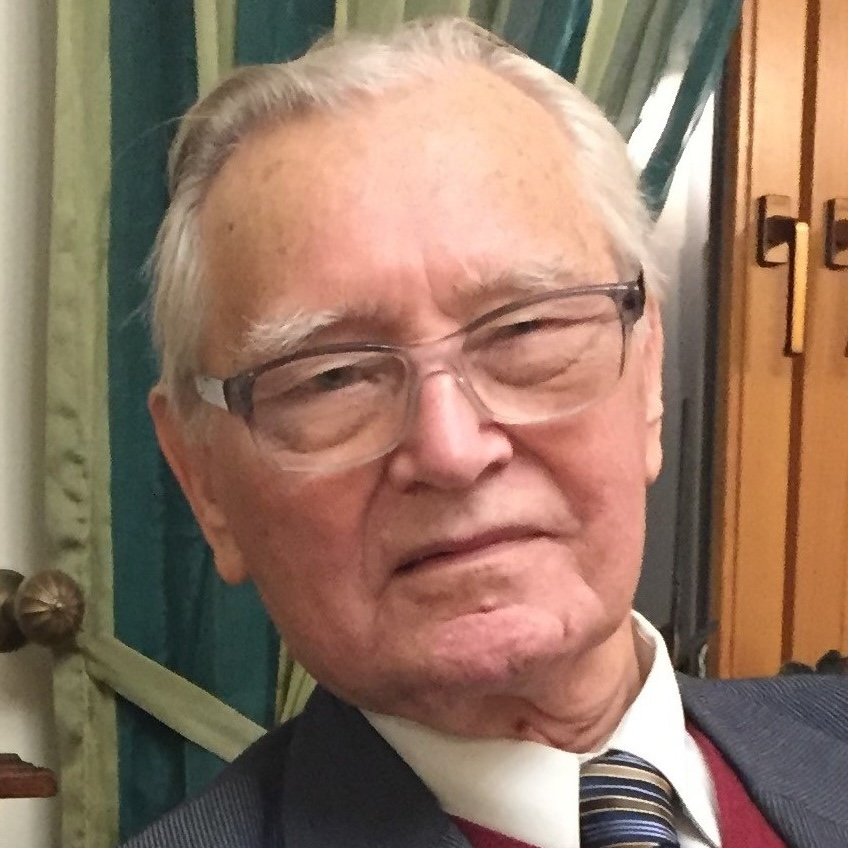
Juliusz Lech Kulikowski
Institute of Biocybernetics and Biomedical Engineering
Polish Academy of Science
Since 1958, the year of an original concept of perceptron by F. Rosenblatt had been published, the interests to computer-aided recognition methods and problems are still alive. After more than 60 years of the development of this research area a question of possible future trends in its development occurs. In this lecture an attempt to answer this question by looking at the problem from a mathematical relations theory point of view is presented. For any given object a computer-aided recognition problem consists, in fact, in indicating an adequate to it similarity class, the object under consideration being to them also similar. The term similarity in this case is interpreted as a property of any two (or more) objects satisfying three basic properties: reflexivity, symmetry, and transitivity. Like the relation Similarity denoting a specific situation existing between some pairs or larger sets of objects and being background of Pattern Recognition – a research area of computer-aided objects recognition, several other binary relations. This can be illustrated the following example: Let it be considered a finite group of patients spending time in a sanatorium. They can be considered as “objects” satisfying the Matching relation, which means that: Any pair of patients is called being “matched” if in some common time-intervals and a fixed room they are subjected to some their individual and proper rehabilitation procedures. All such pairs of patients constitute also, so called syndromes of the relation Matching, as being reflexive and symmetrical. Moreover, the non-transitiveness of the relation follows from the fact that matching A to B and to C can be reported to different times or locations and for this reason matching A to C cannot follow from the former ones. The Matching relation can thus be used, in particular, to investigate the phenomena like e.g. propagation of some infection in large populations. However, our aim is not to describe any of the examples fully, but only to show that the relations-based approach opens new areas of research problems in computer-aided recognition: not only – patterns or – objects, but also – events, states, etc.
Computational Intelligence in Computer Recognition Systems
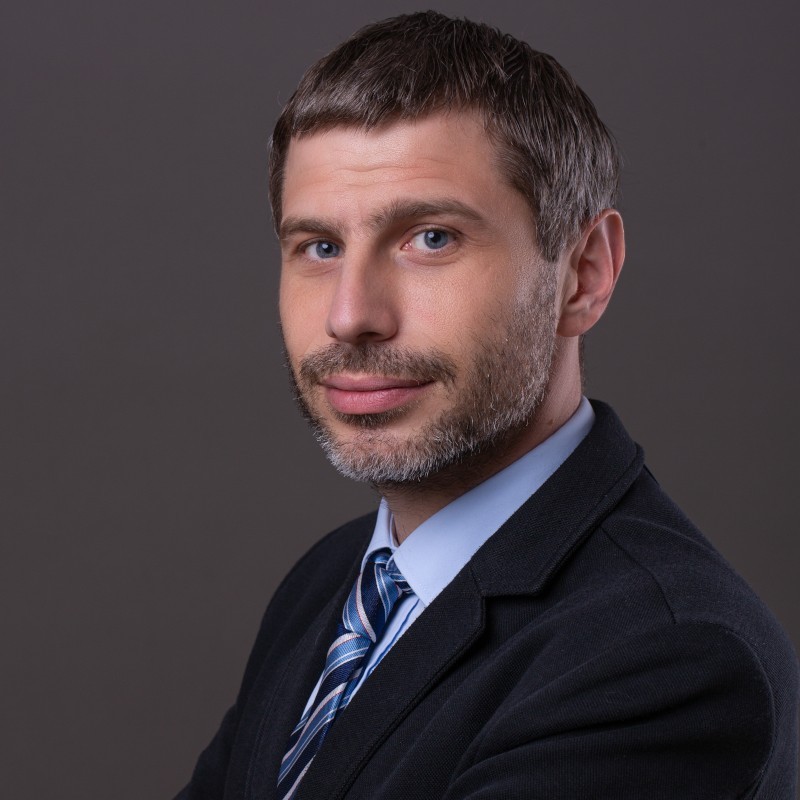
Jan Kozak
University of Economics in Katowice
In this talk, we will explore the power and flexibility of ant colony decision forests, a family of hybrid, nature-inspired learning methods designed to tackle complex and dynamic recognition tasks. The collective intelligence paradigm of ant colonies can be used to allow these decision forests to adapt to evolving data distributions while maintaining high levels of accuracy and robustness. We will discuss the various adaptation mechanisms such as pheromone-driven search and incremental model updating that enable ant colony decision forests to overcome challenges common in real-world data, such as class imbalance, noise, and nonstationary environments. One of the key aspects of this approach is the use of preference-driven measures to guide both the construction and evaluation of the models. By incorporating expert domain knowledge and user-defined preferences, these metrics enable more interpretable results and facilitate fine-tuning performance criteria beyond traditional accuracy-based measures. This perspective is particularly valuable in applications where costs, risks, and contextual constraints play a significant role in decision-making, ranging from anomaly detection in sensor networks to personalised recommendation systems. Drawing on a series of studies and practical implementations from my previous work, I will illustrate how the combination of biologically inspired algorithms with preference-driven evaluation provides an effective framework for building resilient and adaptive computer recognition systems. Real-world examples will demonstrate the effectiveness of these methodologies in coping with diverse data sources and varying operational conditions.
Computational methods in healthcare: A successful case study in ICU
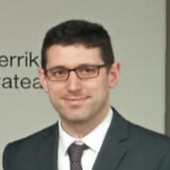
David Nuñez
A predictive model is developed to assist medical professionals in making clinical decisions in Intensive Care Units (ICUs). This model is designed to (a) improve the early prediction of mortality, (b) support more efficient decision-making for high-risk patients, and (c) assess the effectiveness of new treatments or identify shifts in clinical practices. The model is a hierarchical machine learning framework based on Bayesian classifiers, constructed using data from a real-world ICU cohort. It evaluates mortality risk and predicts either the patient's discharge destination if they survive or the cause of death if they do not. The model, called Ensemble Weighted Average (EWA), combines five base Bayesian classifiers into a weighted ensemble using the average ensemble criterion. EWA's performance is benchmarked against other advanced machine learning predictive models. The results demonstrate that EWA surpasses its competitors and offers an additional advantage over majority-vote-based ensembles by providing confidence levels for its predictions. Furthermore, we demonstrate the benefit of locally recalibrating the standard APACHE II-based mortality risk model with new data, even though it proves to be less predictive than the other models.